Call for Remote Dual Certificate Post-Doc Positions in Healthcare Intelligence
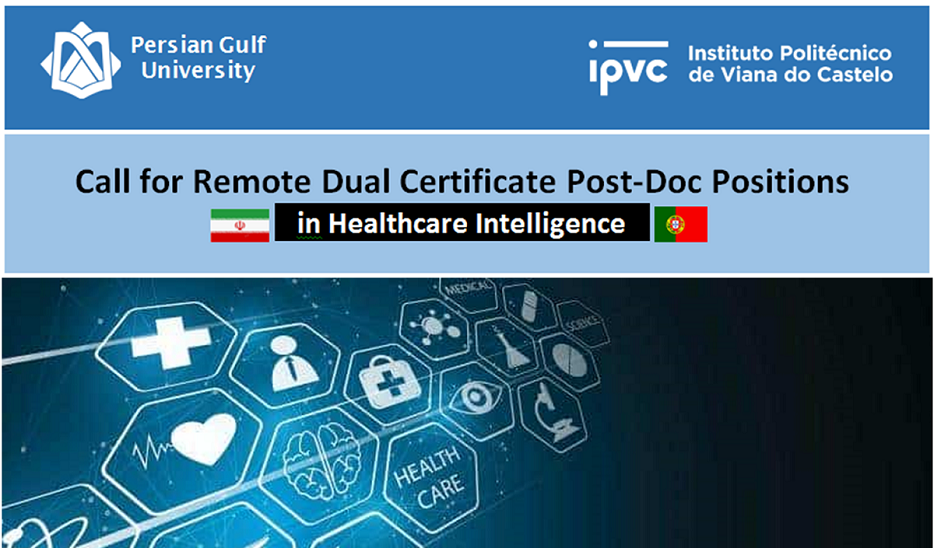
Extended deadline
Download the poster of the call from Here
1. Introduction
Based on the MoU between Persian Gulf University and Instituto Politecnico de Viana do Castelo dated July 16th, 2020:
The Int’l Affairs & Overseas Students at Persian Gulf University announced this call to nominate the candidates for one remote post-doc position in medical image analysis starting in 2022. Two completion certificates will be issued independently from PGU and IPVC.
2. Position and Conditions
1- One Post-Doctorate Position (Code: ICT1)
Title: “Explainable and Domain Adaptive Deep Neural Network for Chest X-Ray Interpretation”
Description: https://pgu.ac.ir/en/news/1088
Supervisors:
Dr. Sara Paiva, IPVC;
Dr. Jorge Esparteiro Garcia, IPVC;
Dr. Andreia Teixeira, IPVC;
Dr. Habib Rostami, PGU;
Dr. Ahmad Keshavarz, PGU

3. Funding and Duration
Duration: 1 to 2 years remotely or partially remote
Funding: Monthly salary plus research grant.
4. Who can apply?
PhD holders in Computer Engineering, Electrical Engineering and other Engineering disciplines familiar with artificial intelligence and medical image analysis can apply.
Competences recommended:
Deep Learning; Image Analysis; Python Programming; A Deep Learning Platform (e.g. TensorFlow, Pytorch)
5. How to apply?
The applicants can apply via email and send the required documents to (ict [at] pgu.ac.ir) before the deadline. Please write ApplicantName_PostDoc as the subject of email.
Extended deadline
The strict closing date of the call is June 1, 2022 (Khordad 31, 1401).
6. Required documents
- Motivation Letter (one page; including the title and code of the post-doc position)
- Recommendations from Supervisor(s)
- CV
- PhD and Master Transcripts
- Competencies Certificates (Recommended)
- Language proficiency proof (Recommended)
7. Completion
Two Scopus indexed publications (one of which in JCR IF Journal) are required to complete the projects, and two individual completion certificates will be issued from each side.
*
*
*
*
More Description on PGU-IPVC joint Post-doc position
Title:” Explainable and Domain Adaptive Deep Neural Network for Chest X-Ray Interpretation”
Overview
Chest radiography (chest X-ray, CXR) is the most commonly performed radiological modality in the world with industrialized countries reporting annually, an average of 238 erect-view chest X-ray images acquired per 1000 of the population [1]. It is estimated that 129 million CXR images were acquired in the United States in 2006 [2]. There are many papers that report that there is significant disagreement between physicians in the interpretation of the same CXR images [5-7]. A report by [3], shows that there is 22% disagreement between the first and second reports of CXR images of children aged less than 5 years. In [4], the authors reported that the proportion of agreement between radiologist and emergency physician reports for normal, congestive heart failure, and pneumonia cases was only 84.3%, 41.4%, and 41.4%, respectively.
Currently, deep learning techniques are applied to a range of problems in science, engineering, and medicine [9]. Since 2012, a form of deep learning technique, called Deep Convolutional Neural Network (DCNN), has been widely used [9]. Because of promising results of Deep Convolutional Neural Networks, recently, deep CNNs have been successfully applied in medical fields [10-13], and especially CXR interpretation [8,14]. Despite wide research studies for using deep learning and especially deep neural network models in the interpretation of CXR images, the clinical usage of these models is very limited due to the limitation of neural networks in 1) adaptation to new domains (different brands of X-ray systems), 2) explaining the function that yields a diagnosis to physicians and 3) reporting all possible findings of the radiographs. Thanks to many public CXR datasets and the development of some domain adaptation techniques in different fields of deep learning, having a domain adaptive model is possible. On the other side, explainable neural networks are a hot research topic with some applicable results.
The purpose of this project is to develop an explainable and domain adaptive neural network model and its software implementation to classify important possible findings in chest x-ray radiographs. Competences required: Deep learning, Image Processing, Software Development
References
[1] United Nations, 2008. United nations scientific committee on the effects of atomic radiation (UNSCEAR), 2008 report on sources and effects of ionizing radiation. http://www.unscear.org/docs/publications/2008/UNSCEAR_2008_Annex-A-CORR.pdf.
[2]F.A. Mettler, M. Bhargavan, K. Faulkner, D.B. Gilley, J.E. Gray, G.S. Ibbott, J.A. Lipoti, M. Mahesh, J.L. McCrohan, M.G. Stabin, B.R. Thomadsen, T.T. Yoshizumi, Radiologic and nuclear medicine studies in the United States and worldwide: Frequency, radiation dose, and comparison with other radiation sources—1950–2007
[3] MA. Elemraid, M. Muller, DA. Spencer, SP. Rushton, R. Gorton, MF. Thomas, et al. (2014) Accuracy of the Interpretation of Chest Radiographs for the Diagnosis of Paediatric Pneumonia. PLoS ONE 9(8): e106051. https://doi.org/10.1371/journal.pone.0106051
[4] Al aseri, Z. Accuracy of chest radiograph interpretation by emergency physicians. Emerg Radiol 16, 111 (2009). https://doi.org/10.1007/s10140-008-0763-9
[5] Quekel, L.G., Kessels, A.G., Goei, R., van Engelshoven, J.M., 2001. Detection of lung cancer on the chest radiograph: a study on observer performance. European Journal of Radiology 39, 111–116. doi:10.1016/s0720-048x(01)00301-1
[6]Y. Balabanova, R. Coker, I. Fedorin, S. Zakharova, S. Plavinskij, N. Krukov, R. Atun, F. Drobniewski, Variability in interpretation of chest radiographs among russian clinicians and implications for screening programmes: observational study BMJ, 331 (7513) (2005), pp. 379-382
[7] M. Young, Interobserver variability in the interpretation of chest roentgenograms of patients with possible pneumonia Arch. Intern. Med., 154 (23) (1994), p. 2729,
[8] Erdi Çallı, Ecem Sogancioglu, Bram van Ginneken, Kicky G. van Leeuwen, Keelin Murphy, Deep learning for chest X-ray analysis: A survey, Medical Image Analysis, Volume 72
[9] O. Russakovsky, J. Deng, H. Su, J. Krause, S. Satheesh, S. Ma, Z. Huang, A. Karpathy, A. Khosla, M. Bernstein, A.C. Berg, L. Fei-Fei, ImageNet Large Scale Visual Recognition Challenge, Int. J. Comput. Vis. 115 (2015)
[10] P. Rajpurkar, J. Irvin, K. Zhu, B. Yang, H. Mehta, T. Duan, D. Ding, A. Bagul, C. Langlotz, K. Shpanskaya, M.P. Lungren, A.Y. Ng, CheXNet: Radiologist-Level Pneumonia Detection on Chest X-Rays with Deep Learning, (2017)
[11] D. Shen, G. Wu, H.-I. Suk, Deep Learning in Medical Image Analysis, Annu. Rev. Biomed. Eng. 19 (2017) 221–248.
[12] J.H. Chen, S.M. Asch, Machine Learning and Prediction in Medicine — Beyond the Peak of Inflated Expectations, N. Engl. J. Med. 376 (2017) 2507–2509.
[13] F. Liu, Z. Zhou, A. Samsonov, D. Blankenbaker, W. Larison, A. Kanarek, K. Lian, S. Kambhampati, R. Kijowski, Deep Learning Approach for Evaluating Knee MR Images: Achieving High Diagnostic Performance for Cartilage Lesion Detection, Radiology. 289 (2018) 160–169. [14] H. Behzadi-khormouji, H. Rostami, S. Salehi, T. Derakhshande-Rishehri, M. Masoumi, S. Salemi, A. Keshavarz, A. Gholamrezanezhad, M. Assadi, A. Batouli, Deep learning, reusable and problem-based architectures for detection of consolidation on chest X-ray images,Computer Methods and Programs in Biomedicine, Volume 185, 2020
Log in to post comments