Call for Iran-Denmark Joint Post-Doc: Smart Grid (Deadline Extended)
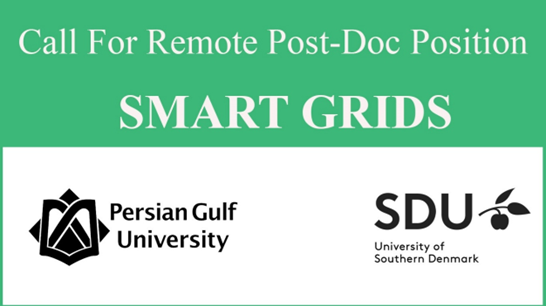
Title
DIGITALLY ENABLED ASSET MAINTENANCE AND MANAGEMENT FOR SMART GRIDS
Download the poster via this link
The Office of International Affairs and Overseas Students at Persian Gulf University announces this call for hiring one joint dual certificate post doc researcher for a project on the digitally enabled asset maintenance and management for smart grids in collaboration between Persian Gulf University and University of Southern Denmark. The details of the call can be found as follows:
Supervisors
Dr. Hamid Reza Shaker, The University of Southern Denmark;
Dr. Rahman Dashti, Persian Gulf University;
Dr. Ahmad Keshavarz, Persian Gulf University;
Funding and Duration
Duration: 1 to 2 years remotely or partially remote
Funding: Monthly salary.
Completion
Two Scopus indexed publications (both of them which in JCR IF Journal) are required to complete the project, and two individual completion certificates will be issued from each side.
Who can apply?
PhD holders in Computer Engineering, Data Science, Electrical Engineering with artificial intelligence and smart grid background can apply.
Qualifications
Good programming skills e. g. Python and Matlab, An outstanding research and publication track record, A solid knowledge of Applied Machine learning and AI
How to apply?
The applicants can apply via email and send the required documents to (ICT [at] pgu.ac.ir) before the deadline. Please write “ApplicantName_PostDoc” as the subject of email.
The strict closing date of the call is July 15, 2023 (Tir 24, 1402).
Required documents
- Motivation Letter (one page; including the title and code of the post-doc position)
- Recommendations from Supervisor(s)
- CV
- PhD and Master Transcripts
- Competencies Certificates (Recommended)
- Language proficiency proof (Recommended)
*
*
*
More Description
Introduction
Digitalization has transformed the world’s power and energy systems and provided many opportunities to effectively address the emerging challenges regarding climate change and increased electrification. Digital data and analytics can help to reduce operations and maintenance costs, improve power plant and network efficiency, reduce unplanned outages and downtime, and extend assets’ lifetime. According to the International Energy Agency (IEA), the overall savings from these digitally-enabled measures would be around USD 80 billion per year over 2016-40. To realize these and enjoy the benefits of digitalization, it is important to develop and deploy new methods for digital asset maintenance and management for the electricity grids.
Research Goal
- The goals of this postdoctoral research are to:
- Improve the current “wait until it breaks” approach to maintenance to a more proactive, predictive, and prescriptive maintenance
- Leverage available digital data e. g. operation data, working environment data, etc to better prioritize asset replacement and plan renovation
Background and/or Theories
Today’s common practice in maintenance in electricity distribution grids is a reactive approach which is accompanied by expensive frequent preventive maintenance on some assets such as transformers and cable boxes. Therefore, the current maintenance practice relies heavily on manual inspection and does not use digital data. Many efforts have been dedicated to improving the current practice, e.g. through fault prediction and location [1]. These methods, although effective, they either not reliable or require data with a very high resolution. To the best of our knowledge, methods that can use the currently available low-resolution data for proactive, predictive, and prescriptive maintenance do not exist. Asset management is more advanced compared to maintenance still heavily relies on expensive manual inspections and assumptions and does not use operational conditions or environmental working conditions such as soil type, proximity to roads with damaging vibrations, etc. These parameters are important to be considered in asset management as they affect the actual lifetime of the assets.
Methodology
In this project, a digital twin will be developed for simulation-based prediction and analysis of faults and critical events in smart grids and the evaluation of possible solutions. This is expected to be carried out in MATLAB or PowerFactory environments. In addition, Machine Learning is expected to be used on the available time series to identify pre-failure symptoms and behavior, allowing us to predict faults at the early stages. Machine Learning will also be used for asset management to combine different available data on environmental working conditions and operation with grid information and to better plan asset replacement.
Log in to post comments